Prologue: The purpose of this article is to document the state of contemporary data science. I am a professional data scientist and machine learning engineer. Two days after I began writing this article, a group letter was published calling for a halt to the ‘out of control’ race to build advanced data science tools. That letter only amplifies the urgency around this topic.
I made an effort here to share relevant perspective and opinion. This is a difficult subject that some people seem to feel very strongly about. It’s made more difficult by the way the technology landscape is changing weekly. My goal is not to answer a plainly provocative question, but rather to share some background I find useful in forming my personal opinion. Hopefully this view from the trenches can help you strengthen your own understanding of what has recently become the frontline in a fast moving global conflict.)
First, the mathematics. Math has been a critical tool in the progress of human civilization for over 20,000 years. From the engineering of great structures to the development of new medical treatments, mathematical thinking has played a crucial role in advancing our understanding of the world and improving the quality of our lives. Today, machine learning – a form of mathematical model – is increasingly being used to solve complex problems and optimize decision-making processes.
This essay explores the potential of machine learning technology. We will consider some popular concerns as to whether this technology could replace human workers entirely. In order to better understand those fears, we will explore the relationship between humans and math throughout history. We will also examine the capabilities and limitations of machine learning in order to predict the impact it may have on our lives.
As we start down this path, let’s recall the words of Galileo Galilei, a pioneering scientist who spent his last decade of life imprisoned for publishing a mathematical proof of heliocentrism:
“Mathematics is the language in which God has written the universe.”
– Galileo Galilei
Estimated reading time: 18-25 minutes
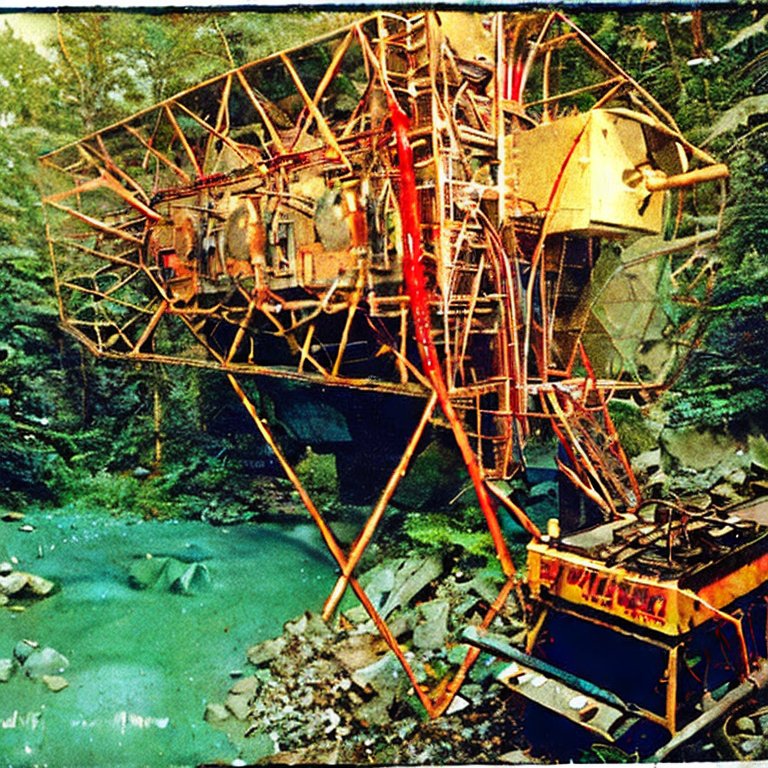
Part I: Math Allows Us Live Our Best Lives
Part I gives a brief summary of the past 100 years of human progress in the areas of Health, Education, Technology, & Environment.
Global life expectancy has increased significantly over the past century, with the largest gains occurring in low- and middle-income countries. In 1920, the global infant mortality rate was estimated to be around 150 deaths per 1000 live births. By 2020, this had declined to an estimated 28 deaths per 1000 live births.
Math has played a critical role in advancing human health and life sciences. For instance, mathematical modeling and statistical analysis have helped researchers to identify trends and patterns in data, enabling the development of new medical treatments. Through the use of mathematical models and algorithms, researchers have been able to better understand complex biological systems and design more effective ways to identify a range of illnesses and conditions. Overall, these advances in scientific tools have had an enormous impact on global health during the past century.
A Self-Sustaining Cycle of Education, Science, and Progress
Access to education and literacy rates have been on the rise, providing more people with the tools to succeed in life. Education has not only helped to reduce poverty and increase economic opportunities but has also led to significant advancements other vital sectors. As a result of this progress, individuals now have higher living standards and improved access to basic needs. This growth has positively affected our individual lives, but also the way we interact with each other. Education is an invaluable resource that can empower individuals, families, communities, and entire nations, providing them a path to a brighter future.
Math, alongside literacy, is the foundation of a successful education. It fosters problem-solving skills, logical thinking, and analytical abilities which are essential traits in many professional fields. Math also provides the basic structure for to support many other subjects, such as science, engineering, and finance.
Technology has Changed Us for the Better
Technological advances of the past 100 years have profoundly remade our world, bringing vast improvements to communication, transportation, and resource accessibility. These developments have greatly increased connectivity and created countless new opportunities for education and economic growth.
Math plays an essential part in driving technology development. Few aspects of our daily lives are untouched by technology, and like it or not, math is what makes this possible. For example, the device you’re using now, the software it runs, and the data network that brought us together… these math based wonders give shape to our lives like never before.
Math and Science Can Save the Planet
There have been major improvements in environmental protection and sustainability over the past century. For example, air and water quality have improved in many areas, and conservation efforts have helped to protect biodiversity and natural resources. Since the mid-20th century, there has been a strong and fast growing movement toward sustainable forest management practices. Organic agriculture has been practiced for centuries, but it was only in the mid-20th century that it was recognized as a distinct farming approach. In recent decades we have witnessed an unprecedented abundance of organic food products, farmers markets, and farm to table restaurants.
Advances in math and science have been crucial in promoting environmental protection and sustainability. For example, statistical analysis and modeling techniques have helped to identify and mitigate environmental risks, while new technologies have helped to reduce waste and pollution. In addition, innovations in areas such as renewable energy and sustainable agriculture have helped to promote more environmentally-friendly practices.
Math & math-based sciences
have greatly improved the
human condition.
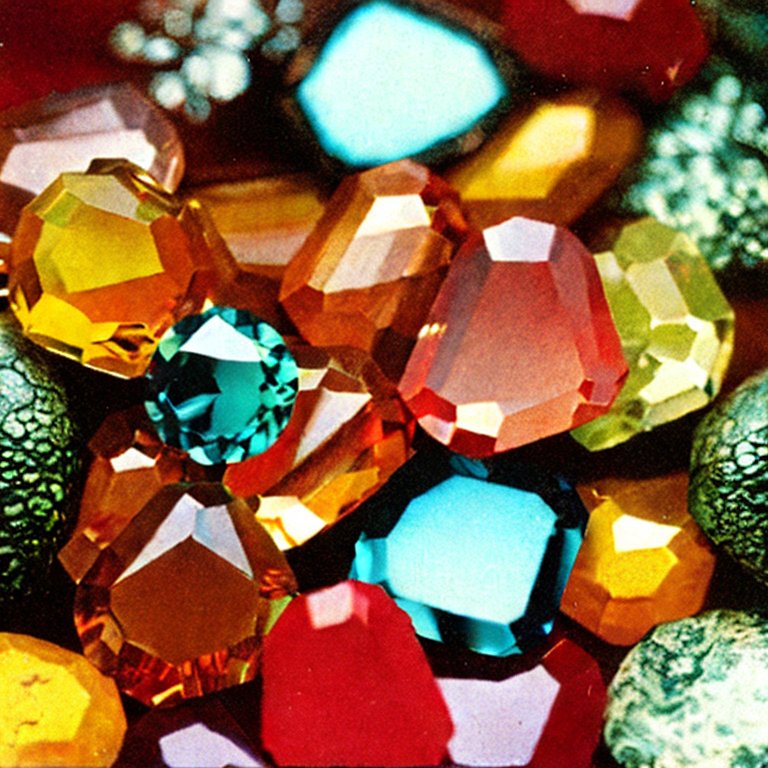
Part II: Machine Learning is Math
Machine learning is a form of artificial intelligence (AI) that allows computers to learn from data and experience without being explicitly programmed. It utilizes algorithms to process data, identify patterns, and make decisions based on the results of the data analysis. Machine learning algorithms are able to detect subtle relationships within large datasets in order to identify trends or anomalies. This can be used to automate decision-making processes and optimize operations in a variety of fields.
Machine learning algorithms are built from complex mathematical models that involve linear algebra, matrix algebra, and calculus. These models are used to analyze large datasets and extract meaningful patterns from them. A machine learning algorithm works by first discovering the underlying patterns in data, then using those patterns to make predictions or classifications on data its never seen before. Linear algebra and matrix math are used to transform and manipulate data, while calculus is used to optimize the model’s performance by adjusting its parameters to minimize a loss function (reducing bad predictions.) Without these mathematical foundations, machine learning would not be possible.
There are many advantages to using machine learning over traditional data analysis, such as speed, accuracy, and the reproducibility of large complex operations. Both unsupervised and supervised machine learning tools are capable of detecting patterns which would otherwise escape human observation.
Machine Learning Capabilities and Limitations
Despite its impressive capabilities, machine learning is not poised to completely replace humans. While it can learn faster than humans, it still has a ways to go in terms of being able to truly understand the context and implications of certain decisions. For example, machines may be able to identify objects in images or recognize speech, but they have difficulty understanding why certain objects may be more important than others.
Furthermore, machine learning is limited by its programming. The tools are only as good as the data they are given and the algorithms they use to process that data. This means that noise patterns in the input data are likely to be present in the output data. Garbage in/garbage out applies directly to the performance of machine learning algorithms.
Humans maintain an advantage when it comes to interpreting complex situations and making decisions based on these interpretations. Machines may eventually become capable of doing this, but for now human judgment is invaluable and irreplaceable in many circumstances.
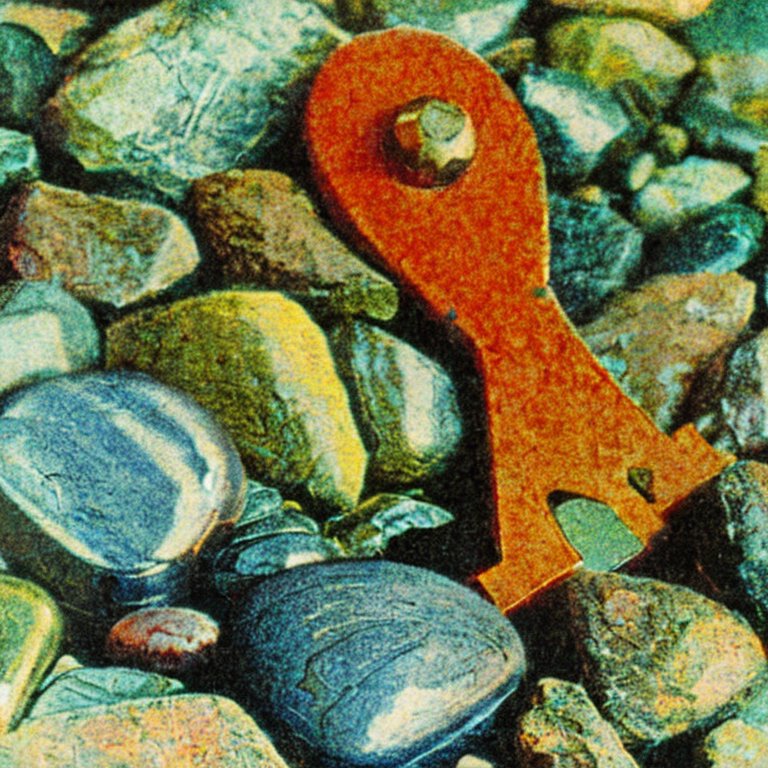
Societal Benefits of Machine Learning Methods
An implicit goal in machine learning practice is to promote human dignity and individual freedom. There are many benefits to what machine learning can accomplish. For example, powerful neural networks could greatly reduce the amount of labor needed in a variety of industries, leading to more efficient and cost-effective operations. This could lead to higher wages and greater job security for high skilled employees. Additionally, machine learning can help lower skill employees learn and adapt to the new economic reality.
Furthermore, machine learning could help automate mundane tasks that would otherwise require human labor or attention. This could free up employees to focus on more important projects while still ensuring that the basic tasks are completed accurately and efficiently. In addition, machine learning systems can be used to detect patterns in data that may not be visible to humans and can provide new insights into business operations or customer behavior.
A Unifying Perspective on Machine Learning Models
The implementation of machine learning technology into our society has the potential to bring with it numerous positive changes. As such, it is important for businesses and governments alike to consider how they might use machine learning technology to maximize benefits for all communities. With the proper implementation of machine learning technology, we may soon see a world where machines take over the most tedious and repetitive tasks, freeing humans to focus on more meaningful pursuits.
“The first peace, which is the most important, is that which comes within the souls of people when they realize their relationship, their oneness, with the universe and all its powers, and when they realize that at the center of the universe dwells the Great Spirit, and that this center is really everywhere, it is within each of us.”
– Black Elk of the Oglala Lakota tribe, 1932
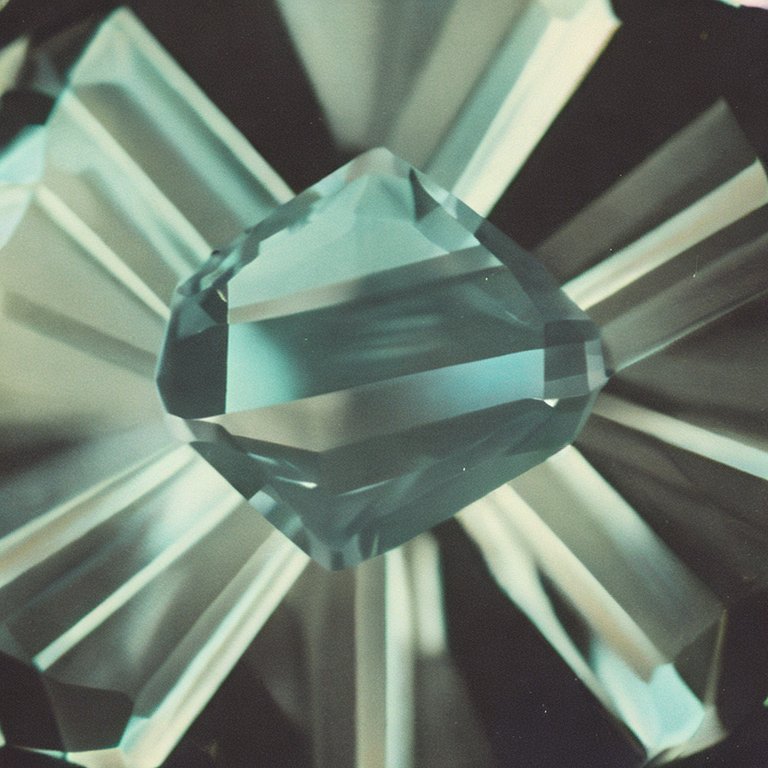
Neural Networks’ Impact On Human Employment
The introduction of various machine learning methods into various industries has raised the question of whether these machines will eventually replace humans. While it is difficult to predict the future, it can be said that machine learning has already had an impact on employment opportunities.
Machine learning is being used in many industries to automate processes and increase efficiency. This has already affected the workforce as many processes are now being handled by machines.
Overall, the debate about the impact of machine learning on human employment opportunities is centered not on whether there will be large changes, but rather on how we can guide those changes in ways that promote human dignity and individual freedom. With careful planning, machine learning could also create new job opportunities for people who are able to adapt and learn new skills.
Machine Learning Algorithms are Expanding to Perform Cognitive Tasks
The automation of machine learning systems to perform cognitive tasks has been a hot topic in recent years. Machine learning algorithms have been proven to perform many complex tasks that were once thought to be impossible to automate.
While it’s true that machine learning can automate some cognitive tasks, such as image recognition or natural language processing, it cannot yet replicate the complexity and creativity that is associated with human intelligence. Machines learning applications are relatively limited in the ability to think critically or come up with creative solutions to problems that lack structured historical data. Furthermore, many complex tasks require subjective decision-making which machine learning applications are not yet capable of.
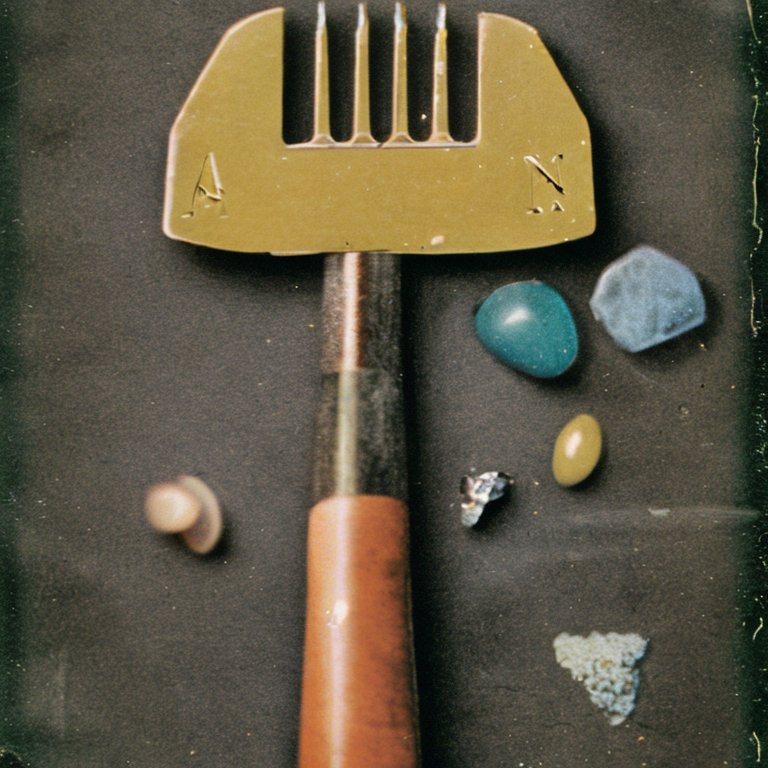
Engineering an Ethical Machine Learning Algorithm
Ethical concerns about machine learning replacing humans are a real concern. This could have a profound impact on society, leading to job losses, poverty, and other issues that could arise if people can no longer find a place in the new workforce. Additionally, machines are not perfect; they can make poor decisions based on faulty data or improper programming.
As machine learning becomes increasingly powerful, there exists the potential that they could become autonomous agents capable of making decisions without human supervision. That development could lead to serious consequences for life on earth.
“Good is positive. Evil is merely privative, not absolute: it is like cold, which is the privation of heat.”
– Ralph Waldo Emerson, ‘Compensation’, 1841
In general, if you want a hammer or other inanimate tool to be ‘good,’ use it build something useful or beautiful. If you want your neural networks to be ‘ethical’, feed them healthy labeled data and train them to do ethical things.
Secure Your Machine Learning System Against Misuse
As machine learning technology advances, so too does the potential for malicious use. With the increasing prevalence of machine learning, it is essential to consider the security implications of this technology. What are some security concerns associated with machine learning and how can they be addressed?
One major concern is that machine learning models could be used to attack systems or manipulate data. For example, an attacker could create a supervised machine learning model to exploit software vulnerabilities or to modify data to manipulate results. Organizations are already using secure coding practices for critical systems, but some cybersecurity systems may not have been tested against a maliciously programmed machine learning model.
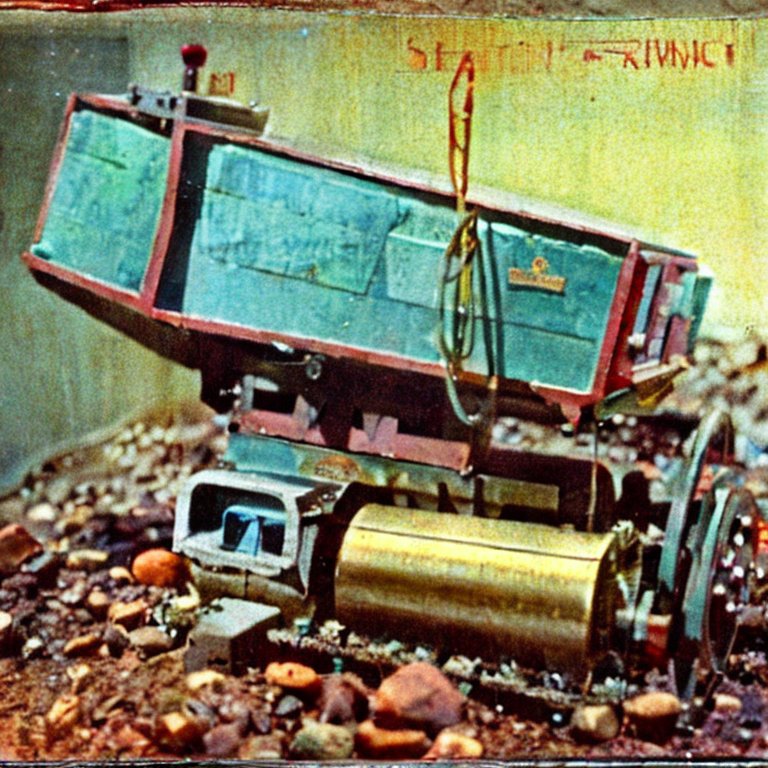
Financial Implications of Automated Data Mining
The potential financial implications of a machine learning-led workforce are vast. If a machine learning model can do the work of humans, it could lead to a decrease in wages and an increase in unemployment. Machines could be cheaper to run than human labor, meaning companies would no longer need to pay wages or benefits. This could have severe economic consequences, especially for those with lower incomes who may not have access to other means of earning a living.
On the other hand, machine learning could create new job opportunities and industries that were previously unimaginable. Supervised machines could also help automate mundane tasks which would free up human workers to focus on more complex and creative tasks or projects. This could potentially lead to higher wages (maybe?) such as the case of an employee who leads a supervised machine to perform complex tasks. This is not the current technical definition of ‘supervised machine learning’, however it’s possible to picture a time when this use case is common.
A Hazy and Uncertain Socio-Economic Outlook
Economic changes always involve tradeoffs and disruptions. Adaptive businesses will thrive, while others will try to gain an exclusive advantage by gatekeeping powerful natural language processing tools. and using machine learning to promote their own view of society. Some players in the industry may abuse their newfound power without even considering the long-term consequences. We are imperfect actors in an imperfect world.
Navigating through the fog of technological advances can sometimes feel like we’re fumbling in the darkness, searching for the faintest glint of light on the horizon. It’s an exciting and uncertain moment. None of us can predict the shape of the economy like in ten years’ time. While much remains uncertain, our history is clear: the politicization of science has never been the path of progress.
“The machine should be a means to an end, not the end in itself. The end is improved human living, and the machine can be used as a tool to help achieve that end. But we must never allow the machine to subvert the ultimate goal, which is to preserve and enhance human dignity and freedom.”
– John Dewey, ‘The Public and Its Problems’, 1927
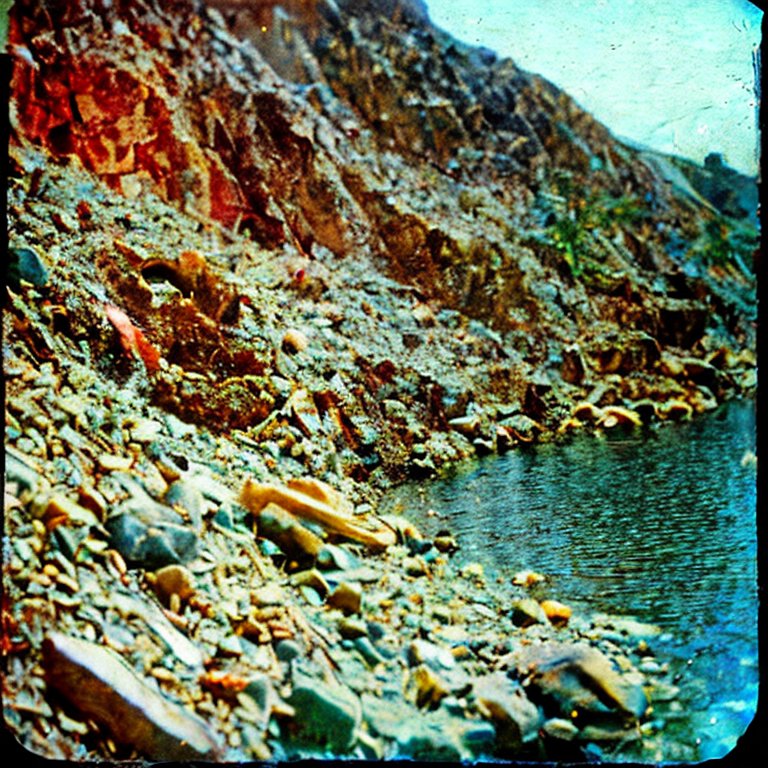
Possible Future Developments in Traditional Machine Learning
Machine learning programs will be an undeniable force in our future. Yet their own future is anything but clear. With new groundbreaking releases now happening every week, the range of possible future outcomes is constantly shifting. These machine learning (ML) advancements aim to boost productivity and make better use of our time, energy, and resources. But they also carry a risk of displacing human intervention. If human intervention can be safely and economically removed from a process, there are engineers working right now to make that a reality.
Although the average human brain is more complex than even the most powerful AI system, AI can surpass us in many specific tasks. For instance, large language models (LLMs) can process text data much more efficiently and accurately than humans. As technology advances, machines are sure to become capable of performing many tasks that currently require human intervention.
Many machines require some form of human supervision, and it will remain essential to check the accuracy of their work, especially in applications that involve natural language or human readable code. Today’s ‘supervisors’ are skilled data scientists who understand the machines well enough to perform quality control. There can be an art and a science to catching a machine’s (often overly confident) mistakes.
As neural network technology advances, we can expect to see an increase in the number of robotic machines taking over physically demanding jobs in factories and warehouses. This trend will accelerate due to cost efficiencies of market scaling. It’s not a new trend but actually dates back to the early industrial era.
Machine Learning is Transformational
Data science has the potential to redefine countless industries, as advanced learning algorithms like deep neural networks gradually replace tasks previously performed by humans. Data scientists are studying ways to optimize these systems, and some are already working to give models the ability to learn independently. Artificial neural networks are incredibly impressive at uncovering hidden patterns high-dimensional datasets. This data mining capability allows software tools to perform tasks with exceptional speed and accuracy.
However, humans are intelligent and adaptable. Technological advances have changed the course of history many times — this is nothing to fear. The rise of machine learning algorithms seems to mark the beginning of a new era, that’s something we can be cautious about without be reactionary. We know the world changes and we know humans can change with it. If an alarmist tells you otherwise, it may be that they’re stoking reactionary fears in order to obscure a different agenda.
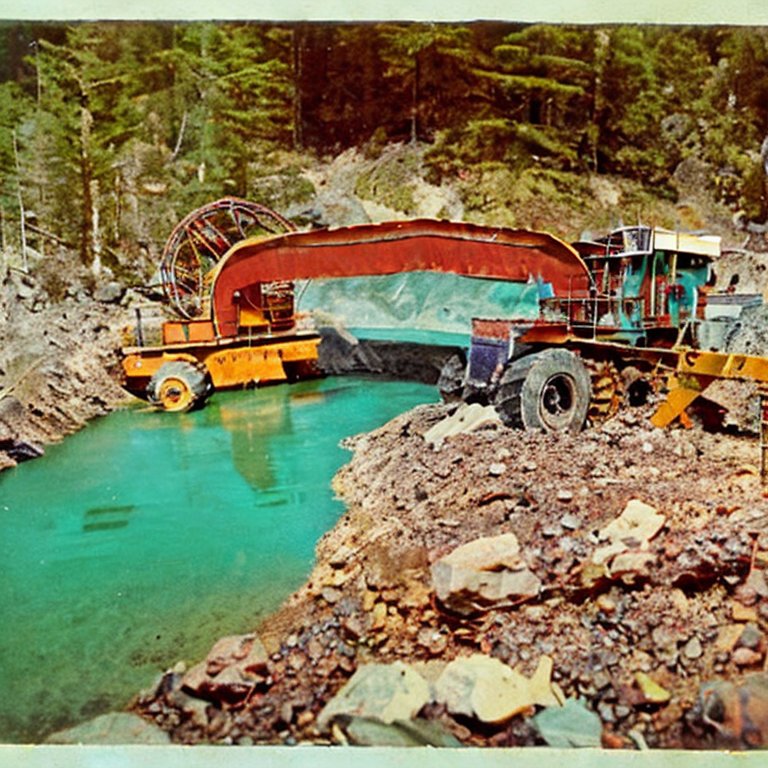
Excessive Regulation Would Unintentionally Benefit the Illicit Informal Sector
There are many reasons why neural network regulations would fail to achieve their stated purpose:
- Biased Enforcement: regulatory action will only tilt the industry further in favor established leaders. We will accidentally create a system where the benefits of AI technology can be controlled by a small number of powerful players. It’s possible this is the direction we’re already moving in. As enforcement actions are shown to be ineffective and politically biased, a grassroots movement will grow around businesses and individuals who choose to ignore unfair rules, partially or entirely. This would foster a black market for illicit AI tools and tool builders. That would not benefit society.
- Regulatory Capture: Regulatory capture occurs when regulators become too close to the businesses they are supposed to regulate, leading to weak or ineffective regulation.
often It’s also important that industry regulations keep up with developments in this field so that proper safeguards are in place to protect against misuse and exploitation. All in all, while machine learning offers great promise for many industries, it must be deployed responsibly with an understanding of its potential downsides as well as its advantages. - Unintended Consequences: Regulations may have unintended consequences that are harmful to the economy or society. For example, regulations designed to protect jobs often end up discouraging private investment, thereby reducing the overall number of employment opportunities.
We can picture how AI regulations might lead us into an undemocratic and two tiered economic system. That would have a profound effect on not only the AI community, but on all of humanity. These tools are too powerful to suppress outright, and that is not what’s currently being proposed. The recent ‘Pause Giant AI Experiments‘ open letter shows us one potential path forward, and the letter carries a great deal of weight due to its having the backing of many large global institutions. Even so, many machine learning experts are troubled by how this letter might legitimize the role of global corporate interests in setting up operating constraints for US private businesses. You don’t need to be a supervised learning algorithm to predict how the regulation of AI research could quickly devolve into all manner of unfair and totalitarian regulations imposed by unelected and unaccountable multi-national bureaucrats. With specific regard to blocking research on a large artificial neural network, this policy solution would create a new and unwelcome market dynamic; one that’s dominated by an elite and exclusive tier of politically connected global industry insiders.
Meanwhile the lower tier of the formal market would be either forced to compete in a type of recreational league, with lesser tools and heavy handed compliance requirements, or they would be driven to the informal sector. Futurist Ray Kurzweil predicted this outcome:
“Artificial intelligence will be the ultimate tool to enable humans to transcend our limitations, but it will also be the ultimate disrupter, creating a two-tiered society where some individuals and organizations can transcend the limitations of biology and the physical world, while the rest of us are left behind.”
– Ray Kurzweil
That’s not the future any of us neural network engineers want. And fortunately there’s nothing forcing us to create such a dark future. I believe we can build something amazing instead.
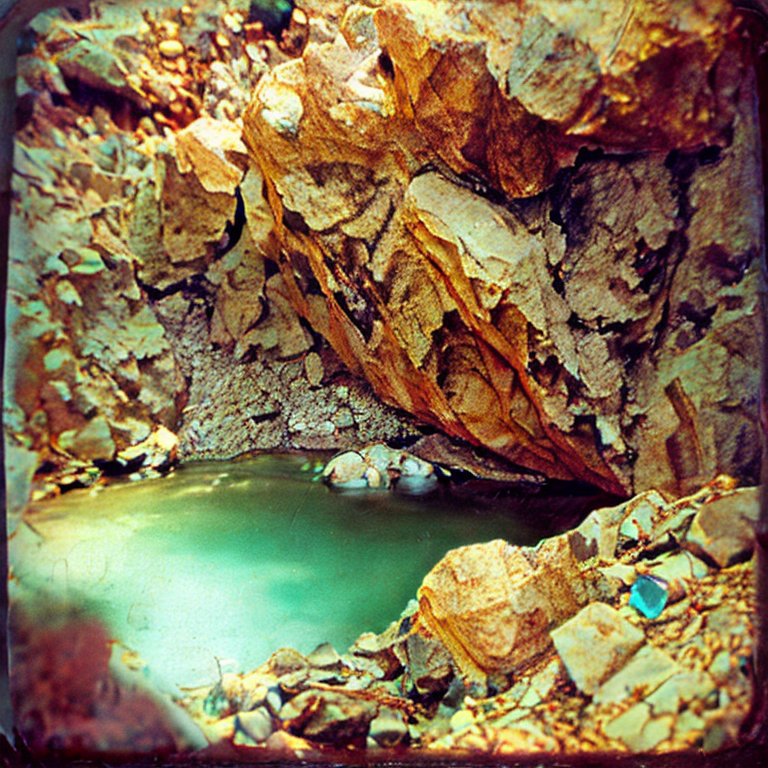
Conclusion: Humanity’s Future Will be Safe & Prosperous
The human brain will fear the unknown more than anything else. There are many unknowns and huge unanswered questions about how far this machine learning applications can go. Ask any data scientists you know how they feel about the changes happening. Neural networks were recently only understood by a small community of research focused data scientists and statisticians. Today’s data scientists have access to profoundly powerful machine learning techniques to perform all manners of complex mathematical calculations. They will tell you math is amazing. Math is fun. Sometimes it might look like magic but data scientists are certainly not magicians. We are people who respect how data mining methods can help us make sense of complex historical data. In that sense, data scientists believe math has always been a force for good… even if math can at times be abused by bad people.
Computer science increasingly involves teaching software to solve problems without human intervention. Machine learning models will play an important role in the future of automation. In conclusion, this represents an opportunity for great progress. If alarmists have made you fear machine learning models, then shame on them. We are blessed to be living through a special moment in human history. Let us not fear when instead we can celebrate the wonder of the world and our short time here together.
Frequently Asked Questions
What’s the Difference Between Machine Learning (ML) & Artificial Intelligence (AI)?
Machine learning and artificial intelligence are two related yet distinct fields of computer science. Machine learning is a subset of artificial intelligence, meaning that while they are closely related, they each have a different scope and applications.
Machine learning engineers of focused on the construction and application of algorithms that can be trained to make predictions. Artificial intelligence, on the other hand, can be achieved through a combination of machine learning models and programming of specific instructions for performing tasks. AI works by creating systems that can imitate human behavior and decision making processes.
Machine learning and artificial intelligence are both closely related fields that deal with teaching machines to perform tasks. Machine learning refers to a type of artificial intelligence where algorithms can learn from unlabeled or labeled training data and adapt to new situations without explicit instructions. In supervised learning a machine is trained using labeled data, and unsupervised learning algorithms find patterns in unlabeled data (eg. clustering algorithms.) Artificial intelligence, on the other hand, refers to any system that can perform tasks that typically require human intelligence, such as perception, reasoning, learning, and decision-making. While machine learning is one way to achieve artificial intelligence, there are other approaches such as rule-based systems or evolutionary algorithms. Therefore, machine learning is best understood as a specific technical approach within the broader field of engineering AI systems.
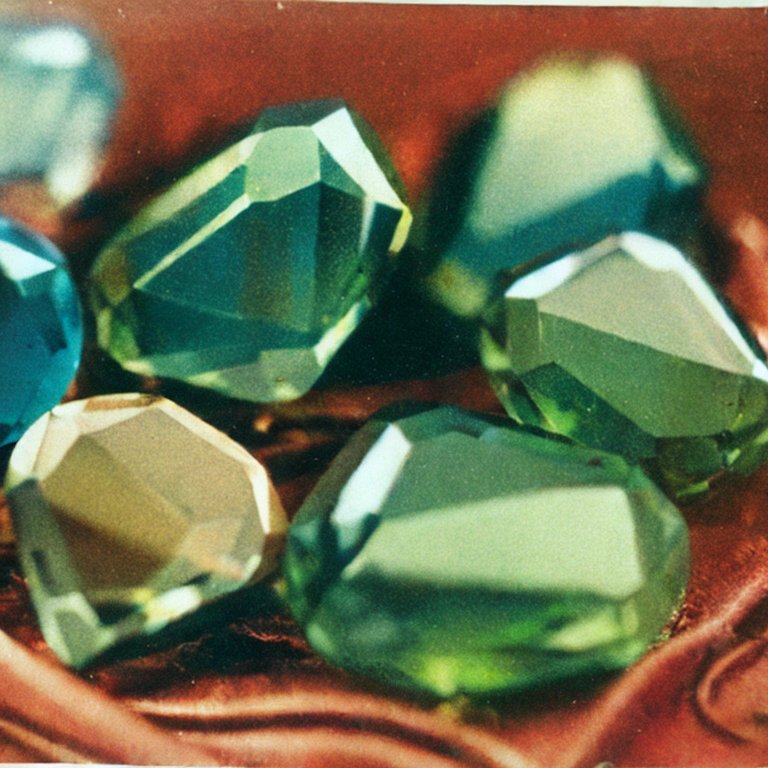
What are Common Applications for Machine Learning Models?
The applications of machine learning are many and deep. In healthcare, AI-powered technologies are being developed to analyze patient data to diagnose diseases more accurately than ever before. Similarly, machine learning can help automate parts of the manufacturing process by recognizing objects within a production line or monitoring machinery for signs of wear and tear. Machine learning can also be used for fraud detection in financial services by identifying irregular transactions or patterns in customer behavior. Finally, it has applications in marketing through personalization techniques such as targeting ads based on user interests or preferences.
As these examples illustrate, machine learning has the power to transform many aspects of our lives – from healthcare to finance to marketing – by automating processes and providing insights into complex datasets that would otherwise be too difficult for humans alone to analyze. With further development and refinement, AI-based technologies could become integral parts of our lives in the near future.
What Are The Potential Risks Of Using Machine Learning?
When considering the potential of machine learning, it’s important to consider the risks associated with it as well. Machine learning is a powerful tool that can be used to automate and streamline many processes, but it’s also not without its drawbacks. In this article, we’ll explore what those risks are and why they should be taken into account when using machine learning.
The first risk of machine learning is the possibility of errors in data sets used to train systems. If there’s any bias or inaccuracy in the training data set, it could lead to incorrect results when the system is put into use. Additionally, if the system isn’t properly tested before deployment, mistakes can go unnoticed until they become serious issues. This can cause problems for businesses that rely on accurate information from their machines.
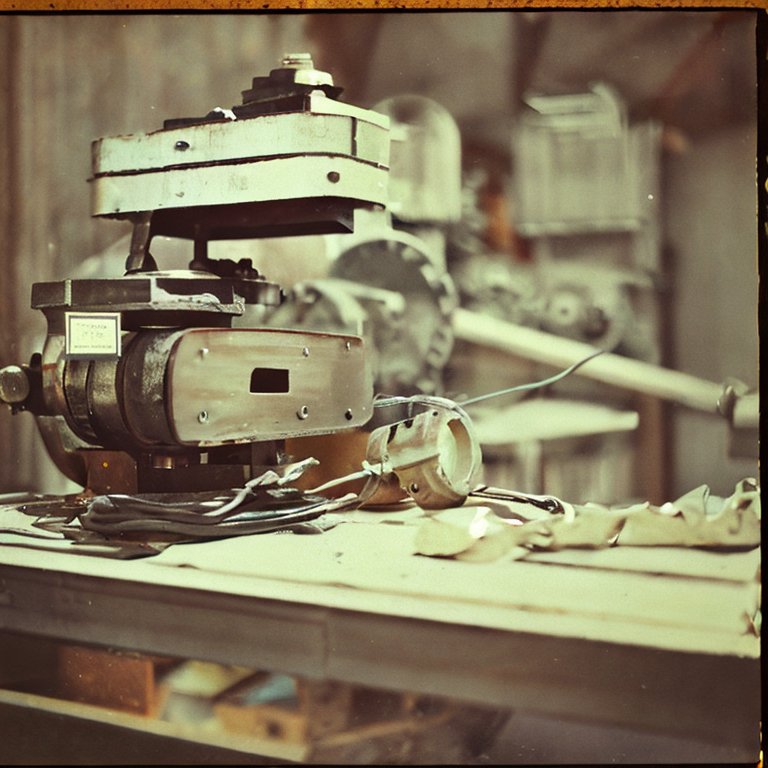
Will Policy Regulations Stop Criminals from Abusing AI Tools?
Machine learning models have the potential for misuse by bad actors. If sensitive data or algorithms are stolen or accessed by cybercriminals, it could have devastating consequences for companies and individuals alike. Given these risks, it’s essential for organizations and individuals to carefully consider how they use machine learning and ensure that appropriate measures are taken to prevent crime. The solution to potential AI crime is not likely to come from public policy or government regulations.
What Are Important Ethical Considerations When Using Machine Learning?
Humans use many types of potentially dangerous tools, and it’s necessary to be safe and responsible when operating tools and machinery. A unique safety consideration for learning machines is related to the privacy of training data. Training a machine learning algorithm requires a large amount of input data. The training data had been anonymized by the time it interacts with the machine learning algorithm. Even so, there may be instances where large platforms can and should do more to protect against sensitive information accidentally becoming public. User data should always be kept safe and secure.
There is the important ethical issue of personal responsibility. Individual AI user might use exploratory data analysis to uncover opportunities to commit crime. That’s illegal and unethical behavior.
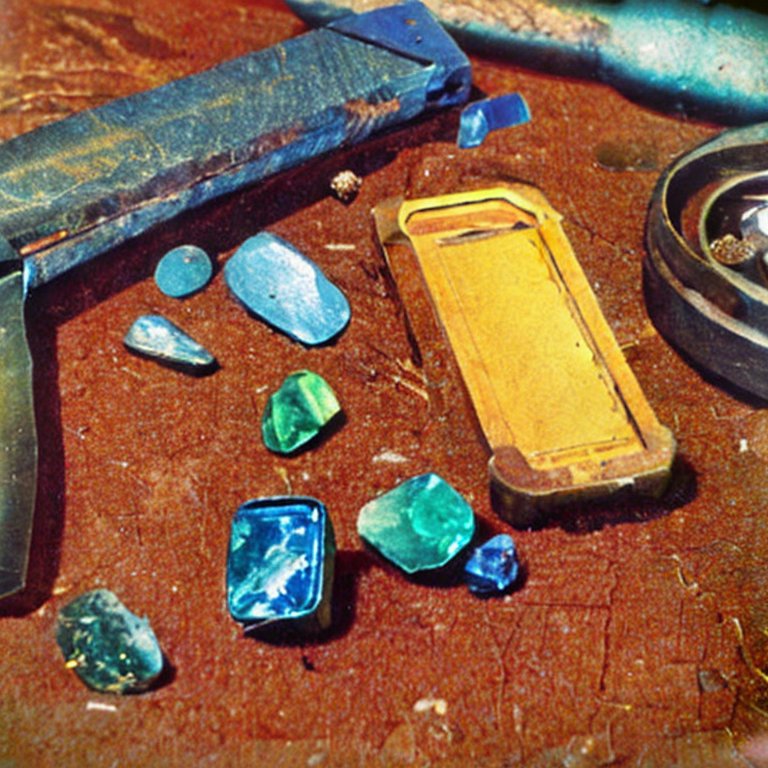
Are You Afraid of Artificial Intelligence?
I do not fear petty ‘harms’ that might result from a machine that generates unpleasant text. I appreciate that unpleasant text can be a problem for some people. That said, the value and utility of this technology is so great as to supersede minor considerations of personal preference. Neither machines or machine users should be encouraged in anti-social behavior.
It’s possible to fear what big data represents to individual freedom. Many brilliant scholars have warned against the ways that big data tools like object detection or image recognition can be used by oppressive governments.
It’s also possible to fear the unknowable logic of different machine learning models. These so-called ‘black box’ models have especially complex internal architecture, and may rely on mind-bending techniques like nested layers of gradient descent calculus. Those models can be hard to understand, especially if they produce unexpectedly spooky outputs. I’m drawn more to learn about these mysteries rather than giving them any power to cause anxiety.
The primary frightening aspect, from my perspective, is that society is unprepared for the economic and cultural changes that could be triggered by GPT5. But that horse may have already left the barn with GPT3. The world has already changed and it’s unclear how we’ll adapt. That’s a tangible issue that we have good reason to be concerned about as we work to understand all types of machine learning. The issue also strikes me as a human problem more than a machine learning process problem.
Often the solution to human problems is to focus on building a positive future. Let’s not shy away from complex tools because they’re unfamiliar and intimidating. Similarly, let’s not diminish outspoken alarmists as “doomers.” There are some legitimate reasons to be alarmed. If the caution flags are disturbing you, I hope you’ll please focus on the things you can control. If you’re concerned about a potentially dark future, in the way I sometimes am, then let’s work together to focus on creating a bright future. We can embrace the present situation and find amusement and joy in the wonder of this life changing technology. It’s a fine time to be alive.
Thank you for reading!
“Science and technology, if they are not to be subjugated to the whims of men, must be guided by a sense of responsibility toward humanity.”
– Alexander Solzhenitsyn, ‘The First Circle’, 1960
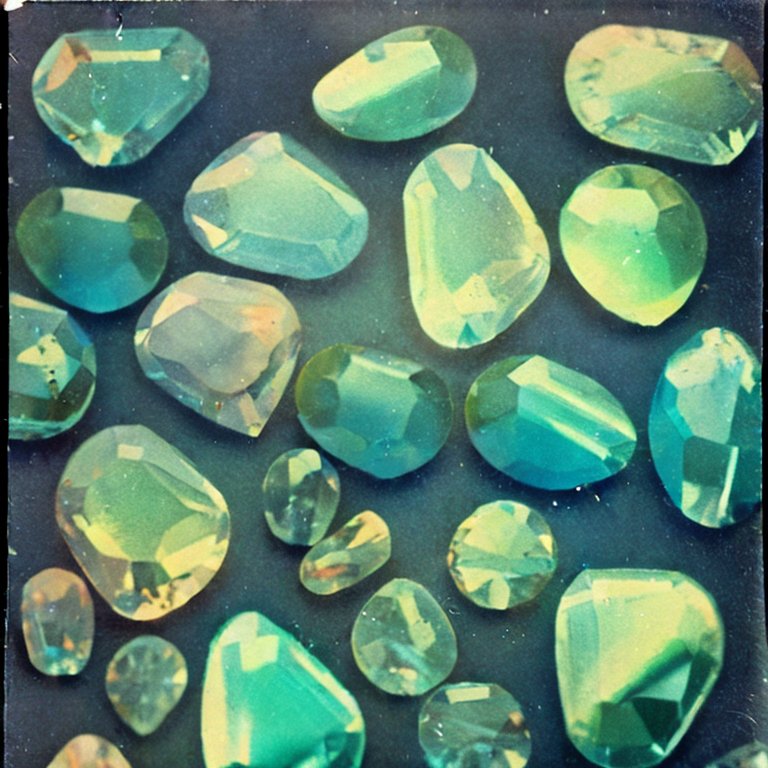